Leveraging Machine Learning for Real-Time Decision-Making in Forex Robot Trading
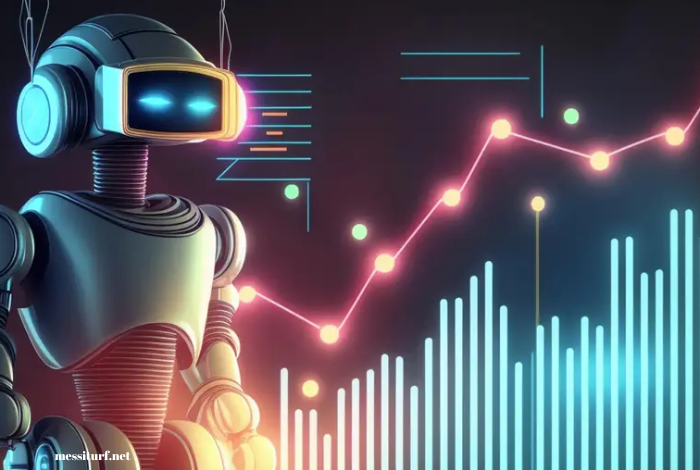
In the fast-paced and highly competitive world of foreign exchange (Forex) trading, making timely and accurate decisions is essential for success. With the advent of machine learning techniques, Forex traders now have a powerful tool at their disposal to analyze market data, identify patterns, and execute trades in real-time. In this article, we explore the role of machine learning in real-time decision-making for Forex robot trading, highlighting how these advanced algorithms enable traders to navigate the complexities of the Forex market with precision and efficiency.
Understanding Machine Learning in Forex Trading
Machine learning is a subset of artificial intelligence that enables computers to learn from data and improve their performance over time without being explicitly programmed. In the context of Forex trading, machine learning algorithms analyze vast amounts of historical market data to identify patterns, trends, and relationships that can be used to make predictions and inform trading decisions.
Unlike traditional rule-based trading systems, which rely on predefined criteria and indicators, machine learning algorithms can adapt to changing market conditions and learn from experience. By continuously analyzing market data and updating their models, machine learning algorithms can improve their accuracy and effectiveness over time.
Real-Time Decision-Making in Forex Robot Trading
Real-time decision-making is a critical aspect of Forex robot trading, where opportunities and risks can emerge and disappear within milliseconds. Forex robots are automated trading systems that execute trades based on predefined criteria and algorithms. By leveraging machine learning algorithms, Forex robots can analyze market data in real-time, identify trading signals, and execute trades with speed and precision.
Machine learning enables Forex robots to make informed trading decisions by analyzing patterns and trends in market data, identifying opportunities, and adapting their strategies accordingly. For example, machine learning algorithms can detect changes in market sentiment, identify emerging trends, and predict future price movements based on historical data.
Pattern Recognition and Predictive Analytics
Two key areas where machine learning excels in real-time decision-making for Forex robot trading are pattern recognition and predictive analytics. Pattern recognition algorithms analyze historical market data to identify recurring patterns, trends, and relationships that can be used to predict future price movements.
For example, machine learning algorithms can detect patterns such as moving average crossovers, support and resistance levels, and chart patterns like head and shoulders or triangles. By recognizing these patterns in real-time data, Forex robots can generate trading signals and execute trades with precision.
Predictive analytics algorithms, on the other hand, analyze historical market data to make forecasts about future price movements. These algorithms use statistical techniques and machine learning models to identify trends, patterns, and relationships in the data and make predictions about future price movements.
By leveraging predictive analytics, Forex robots can anticipate changes in market conditions, identify potential trading opportunities, and adjust their strategies accordingly. For example, predictive analytics algorithms can forecast future price movements based on past trends, economic indicators, and geopolitical events, enabling Forex robots to make informed trading decisions in real-time.
Risk Management and Portfolio Optimization
In addition to pattern recognition and predictive analytics, machine learning algorithms play a crucial role in risk management and portfolio optimization for Forex robot trading. These algorithms analyze historical market data to assess the risk of individual trades, manage position sizes, and optimize portfolio allocations.
For example, machine learning algorithms can calculate risk metrics such as value at risk (VaR), maximum drawdown, and Sharpe ratio to evaluate the risk-return profile of different trading strategies. By optimizing portfolio allocations based on these metrics, Forex robots can maximize returns while minimizing risks.
Challenges and Considerations
While machine learning offers numerous benefits for real-time decision-making in Forex robot trading, it also presents several challenges and considerations. Firstly, machine learning algorithms require access to clean, accurate, and representative data to produce reliable results. Traders must ensure that their algorithms are trained on high-quality data to avoid biases and inaccuracies.
Secondly, machine learning algorithms may be prone to overfitting—the phenomenon where a model performs well on training data but fails to generalize to unseen data. Traders must implement robust validation techniques, such as cross-validation and out-of-sample testing, to mitigate the risks of overfitting and ensure the generalizability of their models.
Conclusion
In conclusion, machine learning plays a crucial role in real-time decision-making for Forex robot trading, enabling traders to analyze market data, identify patterns, and execute trades with speed and precision. By leveraging advanced algorithms for pattern recognition, predictive analytics, risk management, and portfolio optimization, Forex robots can navigate the complexities of the Forex market with confidence and efficiency.
However, realizing the full potential of machine learning in Forex robot trading requires overcoming various challenges and considerations, including data quality, model accuracy, and overfitting. Nonetheless, the integration of machine learning techniques into Forex trading represents a significant advancement in the field of algorithmic trading, empowering traders to capitalize on opportunities and mitigate risks in real-time.